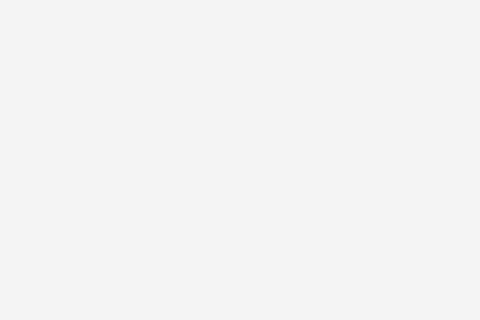
Fusion reactor systems are well-positioned to lead to our upcoming strength preferences in a harmless and sustainable manner. Numerical brands can provide researchers with info on the habits within the fusion plasma, and also beneficial perception around the efficiency of reactor style and design and procedure. Nevertheless, to model the large range of plasma interactions calls for a lot of specialised styles which are not extremely fast enough to provide details on reactor layout and operation. Aaron Ho in the Science and Know-how of Nuclear Fusion team during the office of Used Physics has explored the usage of machine understanding strategies to speed up the numerical simulation of core plasma turbulent transport. Ho defended his thesis on March 17.
The top intention of researching on fusion reactors is to obtain a web strength attain in an economically viable method. To succeed in this doctoral comprehensive examination end goal, considerable intricate products happen to be manufactured, but as these units grow to be a lot more advanced, it becomes progressively vital to adopt a predict-first solution with regards to its operation. This lessens operational inefficiencies and protects the gadget from severe destruction.
To simulate such a process entails brands that might seize the relevant phenomena in the fusion equipment, are correct good enough these kinds of that predictions can be utilized to create trusted design and style decisions and https://www.umflint.edu/hr so are speedily ample to rapidly get workable methods.
For his Ph.D. investigation, Aaron Ho produced a product to satisfy these standards by making use of a product influenced by neural networks. This technique efficiently lets bestghostwriters.net a model to keep both equally speed and accuracy in the price of knowledge assortment. The numerical approach was applied to a reduced-order turbulence product, QuaLiKiz, which predicts plasma transportation quantities brought on by microturbulence. This unique phenomenon stands out as the dominant transportation mechanism in tokamak plasma gadgets. Unfortunately, its calculation is usually the restricting speed point in present tokamak plasma modeling.Ho successfully skilled a neural community product with QuaLiKiz evaluations although by making use of experimental data given that the exercising input. The ensuing neural network was then coupled into a larger integrated modeling framework, JINTRAC, to simulate the core from the plasma product.Operation from the neural community was evaluated by replacing the original QuaLiKiz design with Ho’s neural network model and comparing the final results. In comparison to your unique QuaLiKiz design, Ho’s product thought about extra physics styles, duplicated the outcome to in an accuracy of 10%, and lowered the simulation time from 217 hours on 16 cores to two hrs with a single core.
Then to check the effectiveness for the design beyond the exercising knowledge, the product was employed in an optimization exercising applying the coupled program on a plasma ramp-up situation to be a proof-of-principle. This review presented a deeper idea of the physics behind the experimental observations, and highlighted the good thing about speedy, correct, and in depth plasma products.Last but not least, Ho implies the model could very well be extended for further more programs like controller or experimental style. He also suggests extending the system to other physics brands, because it was observed that the turbulent transportation predictions are no for a longer period the limiting thing. This could further more strengthen the applicability in the built-in design in iterative purposes and help the validation endeavours necessary to thrust its abilities closer toward a very predictive product.